1 min to read
Active Learning for Community Detection in Stochastic Block Models
Is community recovery possible even when D(a, b) < 1 in a symmetric SBM environment?
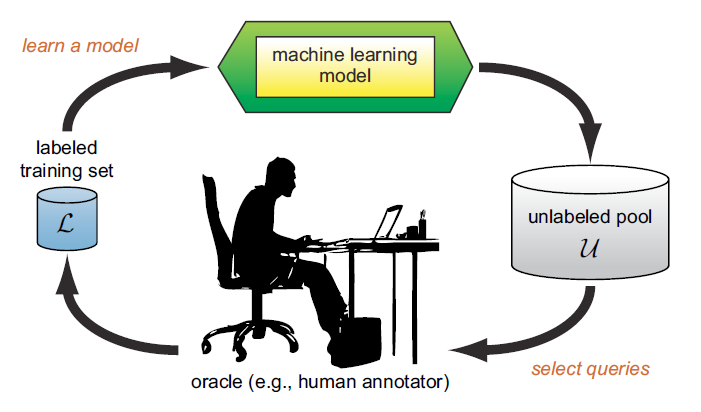
Video
It was recently shown that recovering the community membership (or label) of every node with high probability (w.h.p.) using only the graph is possible if and only if the πΆβππππππβπ»ππππππππ (πΆπ») πππ£πππππππ π·(π, π) =(βπββπ )^2β₯1. An algorithm to obtain a strongly consistent labeling under the assumption π· β₯ 1 is given in [Mossel et al 2016]
In this work, we study if, and by how much, community detection below the clustering threshold (i.e. π·(π, π)<1) is possible by querying (i.e., active learning) the labels of a vanishingly small fraction of carefully selected nodes (i.e., π(π) number of nodes).
This paper suggests a bound on the number of observations (or samples) required as well as an algorithm which specifies which nodes to sample.
Comments