1 min to read
Progressive Layered Extraction (PLE): A Novel Multi-Task Learning (MTL) Model for Personalized Recommendations
Let's go beyond the negative transfer and seesaw phenomenon!
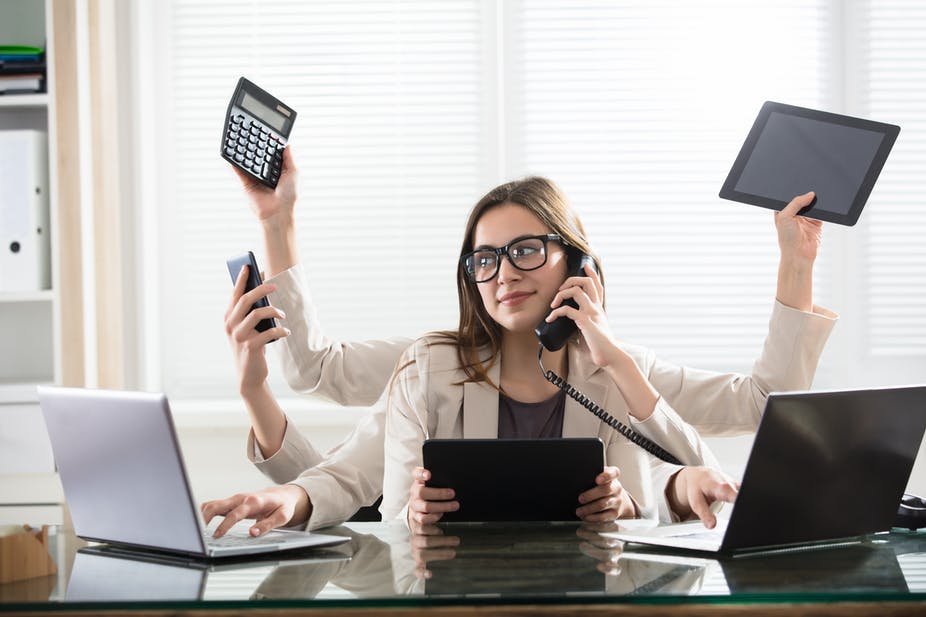
Video
Real-world recommender systems are often loosely correlated or even conflicted, which may lead to performance deterioration known as negative transfer (1: single task, 2: below single task).
When task correlation is complex and sometimes sample dependent, i.e., multiple tasks could not be improved simultaneously compared to the corresponding single-task model, which is called seesaw phenomenon (1: above single task, 2: below single task) in this paper.
Comments